Journal of Financial Planning: July 2014
Cliff Robb, Ph.D., is an associate professor of personal financial planning at Kansas State University. He earned his Ph.D. in consumer economics and personal financial planning from the University of Missouri.
Swarn Chatterjee, Ph.D., is an associate professor of personal financial planning at the University of Georgia. He earned his Ph.D. in consumer economics with a concentration in personal financial planning from Texas Tech University.
Andrew Carswell, Ph.D., is an associate professor of personal financial planning at the University of Georgia. He earned his Ph.D. in urban affairs and public policy from the University of Delaware.
Executive Summary
- This paper examines the performance and impact of portfolios generated from expert stock picks published in three of the leading personal finance magazines. Results suggest that portfolios based on expert stock picks did not beat the market on a risk-adjusted basis during the period of the study.
• As is evident from the analysis, the portfolios generated carry significant unsystematic risk, indicating that diversification could be improved and that holding such a portfolio subjects one to unnecessary risks that are not necessarily rewarded as part of the risk premium.
• Results from the multivariate analyses suggest that after controlling for a number of risk factors, a simple market-indexed, diversified portfolio remained a better option for unsophisticated investors during the Great Recession.
• This study provides further support for individuals’ adopting one of two basic investment
strategies with regard to investments. One strategy is a comprehensive portfolio planning approach, whereby an individual seeks portfolio development and management advice from a professional financial planner to ensure appropriate balancing and diversification. A second strategy is to rely on a simple market-index approach over the long run, rather than trying to keep up with popular investment options.
• Some individuals working with financial planners may choose to pursue hot stocks with a
portion of their portfolio. This paper provides evidence that this strategy does not outperform an index strategy on a risk-adjusted basis.
In today’s market, financial advice is ubiquitous and available in a variety of formats, ranging from a comprehensive financial planner to the far less personalized “expert” stock picks that may be found in magazines, on websites, or in television programs. The underlying logic of such advice is to provide investors with a presumed advantage in terms of asset selection.
It is often generally assumed that following expert advice will yield a superior return relative to those who ignore that expert advice, or relative to those who invest passively with something like an index fund. This logic is somewhat contrary to the logic of efficient markets as detailed by Fama (1965; 1970). One of the central tenets of the efficient market hypothesis is that information is freely available, and it is generally assumed that under most forms of the hypothesis (the weak form exempted) that any publicly available information will already be reflected in asset prices. This further presumes that even sophisticated investors will be unable to beat the market consistently on a risk-adjusted basis without the aid of inside information or a similar nonpublic advantage. This argument is also critical to modern portfolio theory (Markowitz 1952; Fabozzi, Gupta, and Markowitz 2002), which generally argues that investors should seek to construct diversified portfolios designed to match some level of acceptable risk.
Although it is possible to argue the degree to which U.S. markets are truly efficient (strong form versus weak form), and what this efficiency really means for prices overall or how consumers will behave in markets, it is clear that today’s consumers have a wealth of information available to aid them when making investment decisions. Despite the widespread availability of information on value and pricing, evidence from behavioral economics provides strong arguments for why consumers might be drawn to expert recommendations rather than doing their own homework on potential investment options.
In essence, one of the primary problems investors face in dealing with investment decisions might be too much information, coupled with a limited amount of time available to process this information. This can result in consumers essentially failing to make a choice because of information overload (Sethi-Iyengar, Jiang, and Huberman 2003; Agnew and Szykman 2005). In response to the overwhelming amount of information, consumers often seek solutions through the process of heuristic simplification (mental shortcuts).
In this paper, the degree to which reliance on “expert” stock picks provides superior market returns (relative to a simple buy-and-hold strategy using index funds) is assessed.
Literature Review
With the start of each new calendar year, there is no shortage of investment advice available to consumers. This is particularly true in relation to what stocks they should consider adding to their portfolio—for the long term and the short term. This advice comes in the form of websites, blogs, television programs, newspapers, and financial magazines. There is nothing wrong with this advice, as it is often supported by expert analysis; generally, no guarantees are offered (at least explicitly). This is simply another type of information available to consumers.
According to classical economic theory, consumers are able to use all the information available to them when making efficient (or utility-maximizing) decisions. Behavioral economists present a counterargument, wherein consumers may, in fact, fall victim to group psychology or the “wisdom of crowds.” Research suggests that this herding phenomenon is not unique to naive investors. Some theorists highlight this tendency among sophisticated investment managers (Scharfstein and Stein 1990). Keynes (1936) argued that sophisticated investors may be more concerned with the loss of reputation that comes from being contrarian than they are with the potential losses that may arise from relying on group information and behavior, as it often takes a long time for the contrarian view to pan out.
Ideally, individual investors analyze information provided by media outlets, such as financial magazines, and balance this against what the investor already knows about the markets by cross-checking information against a number of alternative sources, including key market fundamentals. However, if individual investors are more likely to simply take the advice provided at face value, allocating investable funds to the securities based solely on the fact that the investments appear on an expert’s top 10 list, there may be concerns over potential herding behavior and long-run outcomes.
Personal finance academics and professionals have referenced mass media as a primary source of information for individual investors who can be categorized as “do-it-yourselfers,” or those individuals who eschew the services of financial planners in the investment process (Certified Financial Planning Board of Standards 2009; Mayer, Zick, and Glaittli 2011).
The Federal Reserve’s triennial Survey of Consumer Finances (SCF) asks questions about the sources of information people use when making decisions about savings and investments. Magazines and print media are given as options in the SCF (Hanna 2011). Evidence from the SCF suggests that people rely on magazines and newspapers slightly more than they use the services of financial planners (Chang 2005).
Bolster and Trahan (2009) found evidence that individual investors followed the recommendations of Jim Cramer (host of CNBC’s Mad Money) in their investment decisions. Karniouchina, Moore, and Cooney (2009) found that the trading volumes of recommended stocks increased on the day following Jim Cramer’s recommendation, thus indicating a recency effect among some investors. These findings are particularly interesting in light of the efficient market hypothesis that suggests common knowledge (or public information) is already incorporated into stock prices, and as such, investors cannot expect to gain an advantage in the market by using knowledge that is widely available.
Earlier work by Hammer and Freedman (2006) articulated potential issues with relying on expert forecasts in the context of Businessweek, noting the difficulties inherent in outperforming market benchmarks on a risk-adjusted basis. This argument is supported by research on individual forecaster performance over time (Bauer, Eisenbeis, Waggoner, and Zha 2003).
Consumers are inundated with a variety of marketing messages within niche magazines. As these periodicals become increasingly competitive, readers have been introduced to a variety of seemingly innocuous forecasts of top 10 lists, geared toward the particular focus of the magazine. This paper examines the potential impact that this informal advice might have on consumer behavior in the area of personal financial planning, with an emphasis on periodicals that specialize in investment-focused information.
Research Methods
For the purposes of this research, three of the most popular personal finance magazines that are broadly available were chosen for review (magazines A, B, and C).1 The three magazines provide a special January issue in which experts pick anywhere from 8 to 12 top stocks for the upcoming year. Each of the chosen magazines carried stock picks in their January issues every year between 2006 and 2011.
Magazine A (recommender 1), magazine B (recommender 2), and magazine C (recommender 3) included 12, 8, and 10 stocks in each year’s portfolio, respectively. Portfolios were developed based on recommended stocks. For example, a portfolio was developed for 2006 and evaluated over a six-year period. A portfolio was then constructed using 2007 recommendations. This portfolio was held for a five-year period and so on. Data on monthly stock returns were obtained from the Center for Research in Security Prices (CRSP) database, which is maintained by the University of Chicago’s Booth School of Business. Returns were used to estimate each firm’s alpha and beta coefficients. Next, excess return was computed by subtracting the firm’s expected monthly return from its actual return. Beta and alpha were computed over the period using three separate regression analyses based on the one-factor capital asset pricing model (CAPM), the Fama and French (1996) three-factor model, and the Carhart (1997) four-factor model.
Research Questions
The data analysis methodology was intended to answer the following three research questions:
- By investing in a portfolio constructed out of financial magazine stock picks, could an individual investor have outpaced the market’s return over the period of the study?
- By investing in a portfolio constructed out of financial magazine stock picks, could an individual investor have outpaced the market’s return on a risk-adjusted basis over the period of the study?
- Do the returns of stocks selected by financial magazines outpace the market over a long-term holding period on a cumulative basis?
Portfolio Performance
The CRSP data used in this study measures monthly total returns for individual stocks using closing prices. To examine the efficacy of investing in these recommended stocks over the long term, a dollar-averaged portfolio (divided equally among each of the stocks that were recommended by the magazines for each year during the 2006–2011 period) was created for each recommender. Returns of the magazine picks in 2006, 2007, 2008, 2009, and 2010 were analyzed over the 2006–2011, 2007–2011, 2008–2011, 2009–2011, 2010–2011, and 2011 periods, respectively. Summary results of the buy and hold portfolios, where all positions were assumed to be held until the end of 2011, are presented in Table 1.
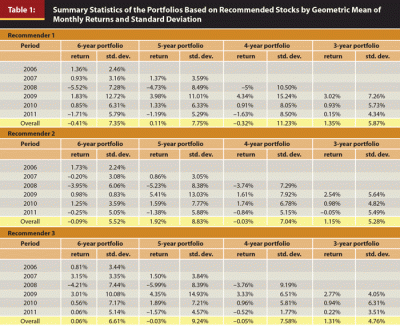
Next, the geometric returns for the portfolios during these periods were calculated. Excess returns (alpha) for the portfolios were examined for the periods over which they were held.
Finally, the risk-adjusted performance of the portfolios was measured using the one-factor CAPM, the Fama and French (1996) three-factor model, and a four-factor model.
Jensen’s alpha or 'α' was defined as:
α = Rp–E(Rp)
where, E(Rp) = Expected return
According to the CAPM, which assumes a single risk factor (market risk premium), the expected return of a portfolio is represented as:
E(Rp) = Rf + β(Rm–Rf)
where, Rp is the expected return of the portfolio, Rf is the return on a portfolio of 30-day Treasury bills or the risk-free rate, Rm is the return of the S&P 500 Index2, and β represents the systematic risk of the portfolio.
For the analysis, beta was the weighted average beta of the individuals stocks in the portfolio.3 After rearranging the terms, the regression equation was generated as follows:
Rp – Rf = α + β(Rm–Rf) + e
Once the values for α and β are estimated, the equation may be rewritten as:
α = Rp–[Rf + β(Rm–Rf)]
where, α becomes the estimate of Jensen’s alpha or the excess return of the portfolio over its expected return.
Fama and French (1996) found that there are factors beyond the market risk premium that explain the excess return of the market. Fama and French proposed a three-factor estimation model for calculating the risk-adjusted return.4 This approach is shown here:
Rp–Rf = α + β(Rm–Rf) + sSMB + h HML + e.
where, Rp–Rf is the excess return of the portfolio over the risk-free rate, whereas Rm–Rf represents the excess return of the market over the risk-free rate, respectively. SMB refers to small minus big; this is the difference in the monthly returns between small and large capitalization stocks. HML represents the difference in the returns between value stocks (high book-to-market ratio) and growth stocks (low book-to-market ratio). The three-factor α, as well as the β, s, and h coefficients, were estimated using an ordinary least squares (OLS) regression on the historical data.
As described in Carhart (1997), a fourth factor was added to account for possible momentum effects. This factor called ‘up minus down’ or UMD represents the difference between the returns of stocks moving up and down in value during a month.5 The four-factor model is:
Rp–Rf = α + β(Rm–Rf) + sSMB + hHML + uUMD + e
The intercept α is the four factor alpha. The monthly estimates for Rf, Rm–Rf, SMB, HML, and UMD were obtained from Fama and French (1996) and the liquidity factors section of the CRSP database.
Results
Table 1 shows the geometric mean returns and standard deviation for the portfolios created based on the recommended stock picks (labeled as recommender 1, 2, and 3). In Table 2, Sharpe ratios6 are shown. These were used to compare risk-adjusted performances for the six-year, five-year, four-year, three-year, and two-year equally weighted portfolios comprising the recommended stock picks with the return of the market.
Table 2 provides a summary of portfolio results. Key findings include:
- During the period 2006 to 2011, the performance of the portfolio based on the stock picks of recommender 3 had the best performance. However, the Sharpe ratios indicate that the three portfolios underperformed the market over the six-year period.
- Recommender 2 had the best risk-adjusted performance over the period 2007 to 2011. The portfolios based on both recommenders 1 and 2 had higher Sharpe ratios than the market.
- Between 2008 and 2011, portfolios created based on stocks of recommenders 2 and 3 outperformed the portfolio constructed out of recommender 1’s stock picks. Portfolios based on recommenders 2 and 3 had higher Sharpe ratios than the market.
- During the 2009 to 2011 period, the portfolio based on recommender 3 performed the best based on the respective Sharpe ratio measures of the portfolios. Interestingly, during this period, all three portfolios exhibited a higher Sharpe ratio than the market.
- Overall, the portfolios based on expert stock picks did not display consistently higher risk-adjusted performance compared to the market, even though there were periods during which a few of the portfolios had higher Sharpe ratios.
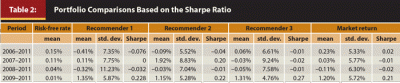
Table 3 provides summary information regarding each portfolio’s risk-adjusted return. Results are broken down into different time periods.
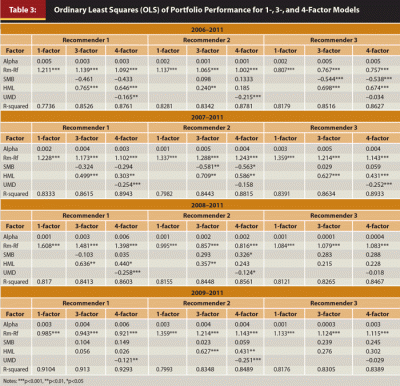
Findings indicate that the alpha was not significant for portfolios created from analyst stock picks in any of the models. The coefficient for the market risk premium (Rm—Rf) variable, which represents beta or the systematic risk of the portfolio in the CAPM, was significantly higher than that of the market for the majority of the portfolios across the periods analyzed. The exceptions to this finding were portfolios based on recommender 3 in the period 2006 through 2011 and recommender 1 in the period 2009 to 2011. This indicates that the expert-based portfolios tended to over expose investors to systematic risk, compared to the market, in most cases.
The HML factor, which represents the difference in return between value and growth stocks, was positively associated with the returns of the portfolios when using the three-factor and four-factor models. This indicates a value tilt in the stock picks of the experts during those periods where the HML factor was significant.
The SMB factor was significant for a number of the portfolios across time periods. When significant, this factor was negatively associated with the selected portfolio, indicating a greater preference for large capitalization stocks. The exception to this finding was the portfolio based on recommender 2 for 2008 to 2011 using the four-factor model (the association of the SMB factor was positive).
The UMD factor was significant in a number of the recommender portfolios across the time periods studied. When significant, the UMD factor was consistently inversely associated with the portfolio in question, indicating that the stocks picked by the recommender did not perform well during the period previous to their inclusion.
Conclusions
This research tested the performance and impact of portfolios created out of stock recommendations provided by experts, as published in leading personal finance and investment magazines. Consistent with the results of many prior studies, this study documents that portfolios created out of stock picks by experts do not beat the market on a risk-adjusted basis over extended periods of time. The absence of alpha indicates that unsophisticated diversification does not yield better-than-market returns when models are appropriately adjusted for risk.
The results of the multivariate analysis suggest that the portfolios’ returns are largely due to greater beta exposure at the market level. A greater exposure to value stocks during most years, and a preference for negative momentum oriented stocks (defined as stocks that do well when the overall market underperforms), also explain the returns in some periods. However, the non-significance of alpha during the period of study is an indicator that the portfolio returns did not beat the market’s return when accounting for the greater market risk exposure of these portfolios.
The present findings suggest that in some periods, experts do provide good advice,7 but in other periods, expert advice is inferior to a simple market index strategy. This provides further support for the argument that even experts are unable to guarantee consistently superior results on a risk-adjusted basis, and reliance on these sources of information, especially magazine tips, may be an ineffective strategy for individual investors who are seeking superior risk-adjusted returns.
The conclusion is one that many novice investors might find to be frustratingly simple. Effectively, a simple market index approach to portfolio development is likely a better option for an unsophisticated investor. This insight held true over both bull and bear market cycles. From the perspective of those advisers and investors who are interested in constructing and maintaining alpha-generating, actively managed types of portfolios, this study is an indicator that even during the period of the Great Recession, a buy and hold indexing strategy would have fared no worse, on average, than a portfolio created out of magazine-based expert stock picks on a risk-adjusted basis.
An obvious limitation of the present analysis is that expert annual stock picks are not necessarily marketed or intended to serve as the basis for an investment portfolio. These are simply securities that the experts believe have some considerable upside potential in the near future, and may be intended as additions to an already balanced portfolio strategy. Evidence from the present analysis indicates that relying solely on such expert recommendations, however, could potentially expose investors to a significant amount of unnecessary (unsystematic risk). This type of risk is not associated with the risk premium presented in modern portfolio theory, and as such, should be avoided.
The present findings indicate that naively selected portfolios created without proper stock selection skills do not consistently outperform the market. If individuals truly wish to adhere to a do-it-yourself investment style, they will be much better off with a simple market index approach over the long run, rather than trying to keep up with popular investment options.
Endnotes
- Initial consideration was given to Money, Smart Money, Kiplinger’s Personal Finance, Fortune, and Forbes. The final list included Smart Money, Kiplinger’s, and Fortune, because these publications carried stock picks consistently in their January issues from 2006 to 2011.
- The primary objective of this paper was to compare the performance of the portfolios developed with the standard market index (the actual S&P 500). The portfolios were also tested against the equally weighted S&P 500 Index (results not shown); results were largely consistent with those presented in Table 3.
- Beta was generated by regressing the monthly returns of the portfolio against the market risk premium and the risk-free rate.
- In the three-factor model, beta was generated by regressing the monthly returns of the portfolio against the market risk premium, risk-free rate, and the Fama-French factors.
- Beta in the four-factor model was generated by regressing the monthly returns of the portfolio against the risk-free rate, the market risk premium, the Fama-French factors, and the Carhart factor.
- Sharpe Ratio=Rp–Rf/Rp, where, Rp = portfolio return, Rf = risk-free rate, Rp = standard deviation of portfolio.
- “Good advice” is defined as that which produces a superior return for investors over the market average.
References
Agnew, Julie R., and Lisa R. Szykman. 2005. “Asset Allocation and Information Overload: The Influence of Information Display, Asset Choice, and Investor Experience.” The Journal of Behavioral Finance 6 (2): 57–70.
Bauer, Andy, Robert A. Eisenbeis, Daniel F. Waggoner, and Tao Zha. 2003. “Forecast Evaluation and Cross-Sectional Data: The Blue Chip Surveys.” Economic Review—Federal Reserve Bank of Atlanta 88 (2): 17–32.
Bolster, Paul J., and Emery A. Trahan. 2009. “Investing in Mad Money: Price and Style Effects.” Financial Services Review 18 (2): 69–86.
Carhart, Mark M. 1997. “On Persistence in Mutual Fund Performance.” The Journal of Finance 52 (1): 57–82.
Certified Financial Planning Board of Standards. 2009. 2009 National Consumer Survey on Personal Finance. Washington, D.C.
Chang, Mariko L. 2005. “With a Little Help from My Friends (and My Financial Planner).” Social Forces 83 (4): 1469–1498.
Fabozzi, Frank J., Francis Gupta, and Harry M. Markowitz. 2002. “The Legacy of Modern Portfolio Theory.” The Journal of Investing 11 (3): 7–22.
Fama, Eugene F. 1965. “The Behavior of Stock Market Prices.” The Journal of Business 38 (1): 34–105.
Fama, Eugene F. 1970. “Efficient Capital Markets: A Review of Theory and Empirical Work.” The Journal of Finance 25 (2): 383–417.
Fama, Eugene F., and Kenneth R. French. 1996. “Multifactor Explanations of Asset Pricing Anomalies.” The Journal of Finance 51 (1): 55–84.
Hammer, Seth, and Ora Freedman. 2006. “Consensus Market Forecasts: Too Risky to Rely on Experts.” Journal of Personal Finance 5 (2): 70–85.
Hanna, Sherman D. 2011. “The Demand for Financial Planning Services.” Journal of Personal Finance 10 (1): 36–62.
Karniouchina, Ekaterina V., William L. Moore, and Kevin J. Cooney. 2009. “Impact of Mad Money Stock Recommendations: Merging Financial and Marketing Perspectives.” Journal of Marketing 73 (6): 244–266.
Keynes, John M. 1936. The General Theory of Employment, Interest, and Money. London: Macmillan.
Markowitz, Harry. 1952. “Portfolio Selection.” The Journal of Finance 7 (1): 77–91.
Mayer, Robert N., Cathleen D. Zick, and Michelle Glaittli. 2011. “Awareness of Retirement Planning Rules of Thumb.” Journal of Personal Finance 10 (1): 12–35.
Scharfstein, David S., and Jeremy S. Stein. 1990. “Herd Behavior and Investment.” American Economic Review 80 (3): 465–479.
Sethi-Iyengar, Sheena, Wei Jiang, and Gur Huberman. 2003. “How Much Choice is Too Much?: Contributions to 401(k) Retirement Plans.” Pension Research Council Working Paper PRC WP 2003-10.
Citation
Robb, Cliff, Swarn Chatterjee, and Andrew Carswell. “More Support for the Old Adage of Staying Cool to Hot Tips.” Journal of Financial Planning 27 (7): 42–48.