Journal of Financial Planning: August 2024
Executive Summary
- Robo-advisers are digital tools that are algorithm-based and provide customers with automated financial advice without human intervention (Huang 2022; Hildebrand and Bergner 2021). Robo-advisers may or may not use artificial intelligence. Artificial intelligence (AI) is a development in technology that is making processing large amounts of data much more manageable than can be done by a human (Jarek and Mazurek 2019).
- This sequential explanatory mixed methods study examined how customers rate trust and satisfaction with robo-adviser services, and it examined the relationship between customer trust and financial services among professionals in the United States who use robo-adviser services.
- The findings of this study showed a lack of education: that robo-advisers exist, how they operate, and how much AI resides in them. There was found to be overall customer trust in both a traditional adviser and a robo-adviser, with reputation of the firm representing a significant positive influence on enabling that trust.
Steve Senteio, D.B.A, CFP®, is a financial adviser in Rhode Island. Steve has research interests in robo-advisers, fintech, and artificial intelligence.
Larry Hughes, Ph.D. is a professor in business administration at Johnson & Wales University and an assistant professor of management at Central Washington University. Dr. Hughes has research interests in leadership, finance, and management.
NOTE: Click on the image below for a PDF version.
A robo-adviser is a finance industry term that can be defined as the automated process of providing decision information without a human interface for the purpose of implementing several elements of wealth management (Chen, Wu, and Yang 2019). Wealth management encompasses financial and investment advice, estate planning, accounting, tax services, and retirement planning.
Robo-advisers are digital tools that are algorithm-based and help customers by providing automated financial advice with no human intervention (Huang 2022; Hildebrand and Bergner 2021). Robo-advisers typically use algorithms, but those algorithms may or may not use artificial intelligence (Thier and dos Santos Monteiro 2022). Artificial intelligence (AI) helps make the processing of large amounts of data much more manageable than can be done by a human (Jarek and Mazurek 2019).
Gaining customer trust in financial technology (fintech) and AI technology in particular can prove to be challenging (Lui and Lamb 2018). A robo-adviser is a subset of fintech and is a newcomer to the fintech landscape; it is not only new to the customer but also to traditional financial firms. Robo-technology is a disrupter in the finance industry (Sironi 2016). Can they be trusted by all users?
This study is important because gaining access to affordable financial advice is necessary to assist in improving financial well-being (D’Acunto and Rossi 2022). This study contributed to the financial technology literature by assessing how customers rate their trust and satisfaction with robo-advisers and human financial advisers. The problem addressed in this study was that trust in robo-adviser technology appeared to be an impediment to its widespread adoption among some demographics of customers seeking financial advice (Guo, Cheng, and Zhang 2019). Trust can be defined as the readiness to be unguarded and to give up power and yield it to the trustor (Cheng et al. 2019). The trust experience is the result of the continuous interaction of a customer’s values, attitudes, moods, and emotions toward a person or an entity (Jones and George 1998). Trust can be interpersonal or institutional. Trust in a robo-adviser could emanate from institutional trust where there seems to be a sense of safety with that institution (Cheng et al. 2019). For example, if one trusts Vanguard as an institution, then it may be likely that they trust their robo-adviser. Additionally, with artificial intelligent chatbots, use of those services has been found to be slow (Nashold 2020). Chatbots are software programs that have conversations in the language of the user (Melián-González, Gutiérrez-Taño, and Bulchand-Gidumal 2021); chatbots that are AI-driven use natural language processing for human–machine interaction and they use machine learning (Doherty and Curran 2019). One of the reasons for slow adoption of artificial intelligence virtual finance assistants is that the customer must disclose considerable personal information to a machine instead of a human (Nashold 2020). Another reason for low trust and therefore slow adoption of artificial intelligence digital financial assistants is that several of the robo-adviser companies are startups/new to the financial industry and do not have the reputation of an established financial firm (Guo, Cheng, and Zhang 2019).
The purpose of this mixed methods study was to examine how customers rate trust and satisfaction with robo-adviser services and to examine the relationship between customer trust and financial services among professionals in the United States who use robo-adviser services.
Literature Review
The robo-adviser came on the scene in 2008 in the wake of the global financial crisis when the U.S. company Betterment launched the world’s first robo-adviser (Huang 2022). The robo-adviser companies Betterment and Wealthfront both began operations in 2008; however, it was not until 2010 when they first offered financial advice to retail investors (Fisch, Laboure, and Turner 2019). Then from 2008 to 2015, 131 more robo-adviser firms were launched (BlackRock 2016).
Robo-advisers managed $870 billion in assets in 2022 and are projected to manage $1.4 trillion by 2024 (Statista 2023). The leading robo-adviser firm (Vanguard Digital Advisor) has $206.6 billion in assets invested (Friedberg and Curry 2022). Robo-adviser growth has been rapid; however, even at $1.4 trillion assets under management (AUM), they still control only a small fraction of the $98 trillion global AUM (Boston Consulting Group 2023). Also, despite their growth, only 5 percent of U.S. investors use robo-advisers; one of the reasons for this slow penetration is that 55 percent of investors with more than $10,000 invested have never heard of robo-advisers (Wells Fargo 2016).
Robo-Adviser Architecture
A robo-adviser gathers information from the customer on their risk tolerance, their investment time horizon, and many other survey questions as part of the process of getting to know the client; then the algorithm within the robo-adviser automatically recommends a portfolio tailored to the specific needs of the customer (Huang 2022). The robo-adviser generally follows a five-step process: selection of the assets to be used, determining the investor portfolio, asset allocation, monitoring and rebalancing, and performance review (Beketov, Lehmann, and Wittke 2018). The portfolio is made up of mutual funds and ETFs from a pool of hundreds and is diversified among equities, bonds, and active and passive investments; when the market causes the customer’s portfolio to deviate from the target parameters, the algorithm detects an out-of-balance condition and either automatically corrects it or suggests that investor reset the portfolio (Huang 2022). Most robo-advisers perform asset allocation, diversification, and rebalancing, and some robos even do tax-loss harvesting (Fisch, Laboure, and Turner 2019).
In the United States, robo-advisers have to register with the U.S. Securities and Exchange Commission and are subject to the same securities laws and regulations as human advisers (Securities and Exchange Commission 2017). Robo-advisers in the United States primarily use exchange-traded funds (ETFs) because of their liquidity, transparency, and low transaction costs, whereas in other places like China, their robo-advisers use more mutual funds that are active and passive (Huang 2022).
A robo-adviser has become a very real alternative to the typical human financial adviser (Alsabah, Capponi, Ruiz Lacedelli, and Stern 2021). The reasons robo-advisers have grown in popularity is that they can provide high value at a reduced cost (Trecet 2019). Robo-advisers can enhance investment selections while at the same time saving on fees (Belanche, Casaló, Flavián, and Loureiro 2023). Robo-advisers reduce cost and improve access because of the lower initial investment, and there is increased transparency (Isaia and Oggero 2022).
The robo-adviser can continuously monitor portfolios, and when there is a deviation to the allocation detected, they can provide automatic rebalancing; some advanced robo-advisers can provide tax-loss harvesting to sell assets at a loss to gain a tax advantage (Bianchi and Briere 2021). Clients are also moving to robo-advisers because their costs in managing a portfolio are lower than their human adviser counterparts (Bianchi and Briere 2021). Preferences for robo-advisers also have to do with some human characteristics that appear in human advisers as analyzed in a study by Foerster, Linnainmaa, Melzer, and Previtero (2017) where 10,000 financial advisers and 800,000 clients were observed; here it was found client traits such as risk tolerance, age, and income only explained 12 percent of the risk they were exposed to (Bianchi and Briere 2021). The financial solution to a robo-adviser is generally in passive investments (i.e., index funds), whereas Linnainmaa, Melzer, and Previtero (2020) show that some human advisers have a bias toward active management.
How Much AI Is in Robo-Advisers?
It is generally assumed by most people, many experts, and researchers that robo-advisers use AI in some way. However, it is estimated that most robo-advisers do not use AI (Deloitte 2016; Thier and dos Santos Monteiro 2022); some use them for sales, marketing, and customer data activity, and the majority of the platforms that do use AI applications are used for portfolio management (Thier and dos Santos Monteiro 2022). AI uses algorithms, but not all algorithms are AI. However, the robo-adviser platforms that do use the more sophisticated AI approaches rely on proprietary algorithms, and they do not make public the details of how they analyze the portfolios or make recommendations (Bartram, Branke, and Motahari 2020).
In a study of 27 German robo-adviser platforms, only about 18.7 percent were found to have live AI applications in place, and most of those applications that are in place have to do with portfolio management (Thier and dos Santos Monteiro 2022). The AI used in these applications vary from AI-based research for stock analysis as well as AI-based portfolio allocation; further differentiation is difficult because most of the data is proprietary and companies are reluctant to share it (Thier and dos Santos Monteiro 2022). Machine learning (a subfield of AI) is prevalent in many robo-advisers, but it is difficult to know how far and to what the machine learning is applied because of company proprietary considerations; there is wide consensus that AI in robo-advisers has not progressed much beyond machine learning (Maume 2021).
In a non-academic research study of five U.S. robo-adviser platforms, the findings showed how little information there was on how AI played a role in their platforms; the findings were not definitive on whether the platforms used AI (de Jesus 2021). By piecing together information from the company website and use of the robo-adviser, the study reported that four of the five used algorithms and four of the five were assumed to be AI-based algorithms but with no description of any level of detail (de Jesus 2021).
Bianchi and Briere (2022) support the findings of de Jesus (2021) that not all robo-advisers use AI, and in fact most use simple procedures and information gathered to construct an optimal customer portfolio; at the same time, the literature lacks the detailed, specific information on the actual use of AI algorithms in robo-advisers.
Algorithms Inside the Robo-Adviser
The algorithms of the robo-adviser typically utilize variations of the modern portfolio theory prescribed by Markowitz (1952) (Bianchi and Briere 2021); the robo-adviser uses mathematical algorithms to help manage the client portfolios in the most efficient way possible based on client preferences as inputs to allocate to investment assets that are not perfectly positively correlated (CFI 2023). In a global sample of (n = 219) robo-adviser systems, a subset of (n = 28) United States and German robo-adviser systems were specifically examined for workflow. The authors found that the workflow was based on some form of the modern portfolio theory approach, and the findings showed that most of the current robo-adviser platforms use the modern portfolio theory (MPT) (Beketov, Lehmann, and Wittke 2018). Furthermore, the robo-adviser platforms, instead of inventing totally new algorithm frameworks for managing portfolios, tend to improve upon or augment the MPT framework for their algorithms (Beketov, Lehmann, and Wittke 2018).
Costs and Fees
Another aspect of a robo-adviser is the cost of financial advice. Some financial institutions require a minimum of $25,000 or more to open an account with a human adviser, but with a robo-adviser, the minimum can range from zero to $5,000 (Bianchi and Briere 2021). The cost for services of a typical human adviser ranges between 0.75 percent to 1.5 percent of assets per year while the cost of a robo-adviser starts at about 0.25 percent and goes to 0.5 percent (Better Finance 2021). The cost of service and ease of access can potentially open access to financial advice for low-income and low-education-level customers through the use of the robo-adviser (D’Hondt, De Winne, Ghysels, and Raymond 2020).
Robo-advisers are able to charge lower fees than human advisers because they have the opportunity to capitalize on economies of scale; a single computer algorithm embedded in a robo-adviser can be used to serve many clients; a human adviser might have 75 to a few hundred clients with the ideal being no more than about 100 (Kitces 2017). The robo-adviser Betterment, on the other hand, has 300,000 clients and 200 employees giving a client to staff ratio of 1,500 to 1; the number of clients served by an adviser in a typical investment firm ranges from only about 50 to 200 per adviser/staff —giving a vivid picture on why the robo-adviser can charge such low fees (Kitces 2017).
Another cost differential is active mutual funds versus passive index funds; generally human advisers are predisposed to recommend the higher-fee, actively managed funds, while robo-adviser platforms typically use the lower cost, passively managed index funds or ETFs (Huang 2022). According to the ICI 2022 Factbook, actively managed mutual funds account for 57 percent of the mutual fund market in the United States (Investment Company Institute 2022). Typical annual operating expense ratio fees for actively managed mutual funds in 2022 were 0.66 percent; for passive funds, fees were about 0.05 percent (Investment Company Institute 2023).
Trust in Robo-Advisers
There are many reasons why people trust robo-advisers. There are also many reasons why people do not trust robo-advisers. Trust in robo-advisers is presented next.
Reasons to Trust Robo-Advisers
Trust in robots depends on several elements including reliability, robustness, predictability, understandability, transparency, and fiduciary responsibility (Sheridan 2019). To integrate these elements, the robo-adviser is dependent on complicated algorithms in the process of advising customers; therefore, trust in algorithms is a key facet of robo-adviser adoption (Bianchi and Briere 2021).
Since there is algorithm aversion that can affect trust in robo-advisers, trust in the algorithm can be enhanced by explaining to the user the processes that drive the algorithm; additionally, trust in the algorithm can be elevated by simply looking at the output of what the results were (Jacovi, Marasović, Miller, and Goldberg 2021). Besides transparency, there are two other intrinsic trust factors that the European Commission on AI identified as necessary to have trustworthy algorithms: agency and human oversight, and privacy and data governance (High Level Expert Group on AI 2019). From an extrinsic trust standpoint, trust in the robo-adviser algorithm can be built up by having technical robustness and safety of the algorithm, being able to interpret the output, and by having accountability and auditability (High Level Expert Group on AI 2019).
Another reason to trust in the robo-adviser and the algorithms behind it is because the humans or the institutions offering the service are themselves trustworthy and reputable (Prahl and Van Swol 2017). For all the reasons to trust robo-advisers, there are reasons not to trust them.
Reasons Not to Trust Robo-Advisers
There are factors that have reduced the adoption of robo-advisers; in fact, the adoption rates of robo-advisers have not reached the levels that were initially predicted (Au, Klingenberger, Svoboda, and Frère 2021).
There is generally a lack of trust in algorithms; 53 percent of respondents in a global trust in technology study were unlikely to trust AI-based robo-adviser algorithms (HSBC 2019). Just 11 percent of the (n = 12,019) respondents said they would trust a robot programmed by specialists to give mortgage advice, whereas 41 percent would trust a mortgage broker (HSBC 2019). Only 19 percent of respondents indicated that they would trust a robo-adviser to make investment choices (HSBC 2019). This tendency to ignore advice that is generated by computer algorithms is referred to as algorithm aversion (Dietvorst, Simmons, and Massey 2015).
In another study by Niszczota and Kaszas (2020), when the experimental study made it known that the performance of the human adviser was equivalent to the robo-adviser, 57 percent of the participants showed preference for the human adviser. And when there needed to be a subjective judgement made, customers thought the algorithms were less effective than the human adviser (Niszczota and Kaszás 2020).
Some studies show that it may depend on who you are as to whether you trust robo-advisers. Bianchi and Briere (2021) show from a sample of 34,000 French savings plan customers that people who were young and male had a higher probability of adopting robo-advisory service. Brenner and Meyll (2020) have results that show customers with larger portfolio sizes favor human advice over robo-advisers.
Theoretical Framework
This study used the trust transfer theory (TTF) framework shown in Figure 1 to evaluate customer trust. TTF describes the mechanism whereby individual A trusts individual C because individual A trusts individual B who in turn trusts individual C; and trust in individuals or groups can also lead to trust in institutions (Cheng et al. 2019). Institutional trust comes from someone experiencing safety because of assurances put forth from the institution (McKnight, Cummings, and Chervany 1998).
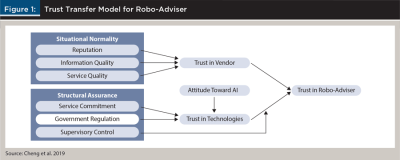
The study had the following quantitative research question and hypotheses:
- RQ1: What factors influence customer trust when using a robo-adviser versus a traditional financial adviser?
- H1: Institutional reputation positively affects a customer’s trust in robo-adviser services and in human adviser services.
- H2: Information quality positively affects a customer’s trust in robo-adviser services and in human adviser services.
- H3: Service quality positively affects a customer’s trust in robo-adviser services and in human adviser services.
- H4: Attitude toward AI positively affects a customer’s trust in the technologies used by robo-advisers (Cheng et al. 2019, 7).
The qualitative question for the study was:
- RQ2: What is the trust experience like for customers in the United States who use robo-adviser technology?
Methodology
The study used an explanatory mixed-methods methodology. The quantitative data were collected first, followed by the qualitative data collection. The quantitative data were gathered using a questionnaire that was uploaded to LinkedIn and administered to working professionals in the United States during the winter and spring of 2023. Respondents were randomly assigned to a robo-adviser experimental group and a human adviser control group. There were (n = 86) total respondents that completed the 40-item survey. The 40-item survey measured eight variables, four of which were used in this study: reputation, information quality, service quality, and attitude toward AI.
The qualitative portion of the study consisted of a 15-to-30-minute semi-structured interview conducted via the Zoom video conferencing application. There were (n = 10) participants who were identified by volunteering at the completion of the quantitative survey to take part in the interviews.
The data was then analyzed using the Statistical Package for the Social Sciences (SPSS) predictive analytics software (version 28). Cronbach’s alpha was run to establish reliability. Alphas were compared to Nunnally’s (1978, 245–246) threshold of .90 or higher for applied research (Peterson 1994). The quantitative data were analyzed using descriptive statistics (Cheng et al. 2019) to examine the frequency, percentages, means, and standard deviation to identify satisfaction and trust construct ratings of robo-adviser users and human adviser users. Once normality was established (Campbell and Stanley 1963), the hypotheses were tested using independent samples t-tests, and the overall model for the primary research question was analyzed using automatic linear modeling.
The recorded qualitative data was transcribed into a text format, input into the Atlas.ti 9 software program and then coded (Cheng et al. 2019). Coding the data enabled the analysis for this phenomenological study because it helped facilitate organizing of the significant participant statements into categories and themes (Creswell and Poth 2018). The code, memo retrieval, and organization report were used to organize all the qualitative data in one convenient package to analyze the phenomenological study.
Results
The results consisted of quantitative findings with the (n = 86) respondents divided into two experimental groups. The qualitative findings were generated from the interviews of the (n = 10) participants.
Quantitative Findings
The robo-adviser user participants comprised (n = 40) the experimental group, and the human adviser user participants (n = 46) were in the control group. Data on five demographic variables were collected: gender, age, education, annual household income, and net worth (Table 1). The majority of the respondents were male (65.1 percent), and the female population represented 30.2 percent of the data. Respondents’ ages were collected in groupings from 18 to older than 64, with the dominant group being those in the 25–34 age cohort (27.9 percent), followed by ages 45–54 (18.6 percent), and ages 35–44 (16.3 percent). Annual household income showed the largest grouping as greater than $150,000 (29.1 percent), and essentially identical to the $50,001–$100,000 grouping (29.0 percent). The separate mean and standard deviation scores for the human adviser and robo-adviser condition are presented in Table 2.
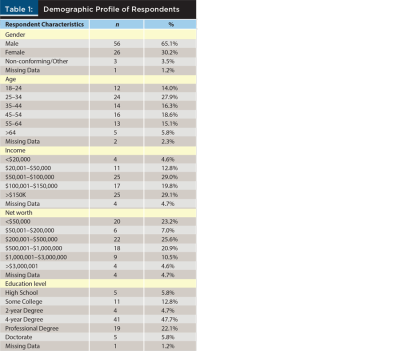
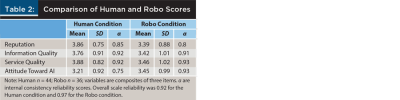
The study variables were measured on a five-point Likert scale. To validate study data normality, the skewness statistics were calculated and there was no evidence of statistically significant skewness in any item or in the composite scores. Also, the Levene’s tests (Table 3) for each reported t-test were non-significant thus providing evidence of normality and homogeneity of variance between scores when comparing both the groups on each composite. Cronbach’s alpha was used to establish the internal consistency reliability of the data collected from the instrumentation in this study. The Cronbach’s alpha for the overall robo-adviser trust score observed in this study was a = .92. The Cronbach’s alpha for the human group was a = .86 and a = .96 for the robo-group. Based on these calculations, the overall robo-adviser study score and the robo-adviser (experimental) group Cronbach’s alpha score could be considered reliable for applied research, while the human (control) group Cronbach’s alpha could be considered reliable for basic research; a > .90 is appropriate evidence of reliability for applied research (Nunnally 1978).
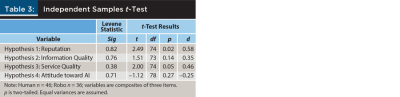
Hypothesis Testing
An independent samples t-test was used to test the four hypotheses of the study. This test includes the Levene’s statistic, which assesses the homogeneity of variance between the robo group and the human group on each variable. If the test is non-significant, then there is homogeneity of variance, and it provides evidence that the independent samples t-tests for this study met the assumption of normality—which did occur for this study.
Two of the four hypotheses for the study research question were determined to be statistically significant: reputation and service quality. Hypothesis 1 states that institutional reputation positively affects a customer’s overall trust in robo-adviser services and in human-adviser services. The third hypothesis states that service quality positively affects a customer’s trust in robo-adviser services and in human adviser services.
Automatic Linear Modeling
The primary quantitative research question leading to this study stated, “What factors influence customer trust when using a robo-adviser versus a traditional financial adviser?” The above analysis revealed support for two underlying hypotheses related to reputation and service quality. But to address the overarching question, automatic linear modeling (ALM) was employed to model the target variable of overall trust and the linear relationships between each of the sub-components and the relevant demographic variables. Given the sizable pool of potential predictors explored in this study, a more robust modeling tool was necessary (Yang 2013). ALM is a tool in IBM’s SPSS that allows these complex calculations to be more readily computed (Oshima and Dell-Ross 2016). The four continuous predictors were reputation, information quality, service quality, and attitude toward AI, and the three categorical variables of human versus robo, age, and net worth were selected in order to observe the impact on overall trust.
The overall model was statistically significant with all four predictors showing as contributing to the overall model fit (F = 8.47(4,66), p < .01). The ALM model did not recognize that age or net worth, significant in the other analyses, carried significant weight in this analysis. In other words, regardless of age, experience with a robo-adviser, or net worth, it was the adviser’s firm’s reputation, service and information quality, and their attitude toward AI that “mattered” in the manifestation of trust. Figure 2 depicts the weights of the significant predictors on the dependent variable of overall trust. In response to the question, “What factors influence customer trust when using a robo-adviser versus a traditional financial adviser,” the answer is that all four of the hypothesized factors matter to some extent, with attitude toward AI contributing the strongest weight.
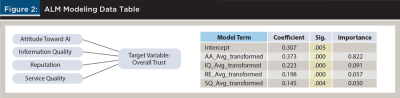
Qualitative Findings
The trust theme was a major finding in this study with the trust experience category having a big influence on the participants trusting the robo-adviser or human adviser. One participant said that the experience would be good if they knew the adviser was looking out for their best interest. Reputation of the firm or the adviser was another significant category under the trust theme, and this had connectedness with the quantitative portion of the study, which measured reputation as significant in influencing trust. One participant said this about reputation: “So, I think what I wanted and who to work with—someone who was established, who had a good reputation, and who was being used by people that I respect and perceived to be abundant and wealthy.”
The functionality theme was another major qualitative finding. This theme was dominated by the convenience category. One participant who uses both a robo-adviser and a human adviser exemplifies the functionality theme when they said: “I feel like I have a little bit more control with the robo-adviser because if I want to add an extra $75 this month, I can do that fairly easily.” And several participants think the robo-adviser is more time efficient. They liked that they did not have to spend the extra time communicating with a human adviser for their desires, and it lowers the effort of interaction. Another convenience of a robo-adviser that participants liked was that they are readily available and at any hour of the day, seven days a week. The robo-adviser “does not sleep or go on vacations.” Participants also liked the auto-rebalancing feature of a robo-adviser. The opposite of convenience was the complexity category that was exemplified by one high-net-worth participant that would only think of working with a human because of their complex financial transactions.
The education theme was a third major qualitative finding. There were three components to the education theme, but for the sake of time, I will just talk about the one issue that crossed over with the quantitative findings in the literature and that is the lack of awareness that robo-advisers even exist. The best example of this in this study was described by one participant when they said that if they knew more about robo-advisers, they would probably invest in them. In fact, they want to find out more information about them.
Discussion and Implications
Both the robo-adviser experimental group and the human control group had an overall positive trust experience from a quantitative and qualitative perspective. Reputation was one of the significant factors in influencing trust both quantitatively and qualitatively.
Convenience of the robo-adviser can be viewed from two perspectives—through the eyes of the vendor and those of the customer. The vendor can access a much larger customer base at decreasing marginal costs. The customer has access to their investments day and night, they can make changes easily, and, finally it is deemed as more time efficient than dealing with a human adviser. A human financial adviser can leverage the technology of a robo-adviser by enabling clients to use a robo-adviser to help manage their investments; however, when it comes time for complex scenarios or explanation of market dynamics, the human adviser can be available for consultation. So, routine tasks like increasing a monthly contribution to a Roth IRA or investment threshold re-balancing can be handled through robo-adviser technology, but when it comes to issues such as how much or whether to convert the traditional IRA to a Roth IRA, the human adviser would step in to answer those more complex questions. Another aspect of robo-adviser technology that could be leveraged by human advisers would be the ability to rapidly uncover the right investment opportunities at the right time through machine learning; this can be done much faster and with more accuracy than a human adviser.
There were four theoretical implications that flowed from the findings of this study. First, it is believed that the current research in this study is the first to explore the robo-adviser education theme in a phenomenological study. The education theme emerged from the interviews in the qualitative portion of this study in three ways. First, the qualitative data in this study supported the quantitative information in literature that there is a lack of knowledge that robo-advisers exist (Brown 2017). Secondly the qualitative interviews uncovered that for those who have heard of or even used robo-advisers, there is a lack of understanding on how they operate. And lastly, this study highlights and extends the research on how much or if robo-advisers use AI in their operation. It is estimated that currently most robo-advisers do not use AI (Deloitte 2016; Thier and dos Santos Monteiro 2022); yet in compiling the literature for this study there is widespread misunderstanding that robo-advisers automatically incorporate AI.
The second theoretical contribution involved extending the research into how complexity of the financial situation influences the trust in human advisers versus robo-advisers. The findings from Northey et al. (2022) show that consumers are accepting of information from both human and robo-advisers when the amount invested is low; when the amount invested is high—which can be interpreted as higher complexity—consumers are less likely to invest in an AI-enabled system like a robo-adviser. Many robo-advisers just limit themselves to portfolio management and do not do retirement planning, estate planning, or insurance cases (Fisch, Laboure, and Turner 2019). This study corroborated this literature and took it a step further with the data from a high-net-worth participant who not only needed the complex capabilities of a human adviser but also wanted a one-stop-shopping approach for their wealth management where all the solutions reside in one office entity.
The third theoretical contribution extends the research on the reputation trust factor from a qualitative perspective. From a quantitative perspective, this study confirmed the hypothesis that reputation of the firm positively influenced trust in both the human and robo-adviser. Cheng et al. (2019) and Kim, Xu, and Koh (2004) also show quantitative results that reputation of the firm or institution positively influence customer trust of the service or technology. The qualitative data in the current study on reputation validated the qualitative comments in Cheng et al. (2019) on reputation of the firm from a corporate level being important in trusting and using the robo-adviser. The current study extended research on reputation by adding qualitative data on the reputation from a peer-to-peer perspective—the customers trusted the adviser service because their friend or spouse used and trusted that service.
The final theoretical contribution extends the research on the artificial intelligence trust factor. Although attitude toward AI (AA) proved not to be significant in the independent samples t-test of the current study in positively influencing trust in the robo-adviser, it did prove to be significant in the ALM test. The ALM test does have greater flexibility in detecting predictor influences and interactions between variables. This difference in outcomes inside this study is also replicated in the sparse body of literature on AA’s influence on trust. Cheng et al. (2019) showed that AA was significant in its influence on trust in robo-advisers, while Kaplan, Kessler, Brill, and Hancock (2023) had results from a meta-analysis that AA did not demonstrate significance to trusting in AI. On the other hand, the qualitative data in the current study actually showed a negative influence on trust in the robo-adviser. The results are mixed and require further exploration.
Limitations
There may not have been enough respondents to take part in the study, thereby affecting power, validity, and reliability of the results. Despite a 73-day data collection period, and although the quantitative sample size did not achieve the design goal, the data did prove to be normal and reliable. A limitation for respondents was the amount of visual exposure they had to the robo-adviser output to enable assessing information quality. Had there been more detailed robo-adviser output than just the portfolio allocation diagram, there might have been a different result in how information quality affected trust.
Another limitation of the study is that, initially during the proposal process, it was assumed that all robo-advisers used artificial intelligence; however, the literature review development uncovered that not all robo-advisers incorporate AI. Fortunately, the survey instrument and the interview instrument incorporated enough flexibility that the knowledge of whether the robo-adviser had or did not have AI incorporated did not appear to be a factor.
The risk tolerance questionnaire instrument in this study was limited to just one question from the validated 13-item and five-item risk questionnaires from Grable and Lytton (1999). This was done due to the limited capability of Google Forms to sum risk score totals in real time during the questionnaire process. The single-item questionnaire was deemed a representative evaluation of the customer risk tolerance for the purposes of presenting an appropriate allocation for the vignette and did not appear to affect the evaluation of customer trust in the study.
Future Research
The current research in this study found relationships of varying statistical significance, namely attitude toward AI and dimensions of customer trust. With all the recent attention on AI highlighted by cutting-edge technologies such as ChatGPT, OpenAI, and driverless cars, this is an area worthy of continued future research. This study recommends that future research examines AI trust factors using different constructs and scale items.
Additionally, it should not be assumed that all robo-advisers have equal applications of AI. Therefore, future research on the topic of artificial intelligence in robo-advisers should also be conducted to test customer trust and satisfaction with rules-based, machine-learning-based, and deep-learning-based robo-advisers.
Conclusion
The findings of this study showed a lack of education: that robo-advisers exist, how they operate, and how much AI resides in them. There was found to be overall customer trust in both a traditional adviser and a robo-adviser, with reputation of the firm representing a significant positive influence on enabling that trust. A robo-adviser showed that it can be a trusted component of financial advising. A human financial adviser can leverage the technology of a robo-adviser by enabling clients to use a robo-adviser to help manage their investments. When a complex financial situation is encountered such as retirement planning, alternative investments, tax planning, or estate planning, at the moment there is no substitute for a human adviser. There will always be a need and a demand for a human adviser.
Citation
Senteio, Steve, and Larry Hughes. 2024. “Customer Trust and Satisfaction with Robo-Adviser Technology.” Journal of Financial Planning 37 (8): 86–101.
References
Alsabah, Humoud, A. Capponi, O. Ruiz Lacedelli, and M. Stern. 2021. “Robo-Advising: Learning Investors’ Risk Preferences via Portfolio Choices.” Journal of Financial Econometrics 19 (2): 369–92.
Au, Cam-Duc, L. Klingenberger, M. Svoboda, and E. Frère. 2021. “Business Model of Sustainable Robo-Advisors: Empirical Insights for Practical Implementation.” Sustainability (2071-1050) 13 (23): 13009–13009. https://doi.org/10.3390/su132313009.
Bartram, Shonke, J. Branke, and M. Motahari. 2020. “Artificial Intelligence in Asset Management.” CFA Institute August. www.cfainstitute.org/en/research/foundation/2020/rflr-artificial-intelligence-in-asset-management.
Beketov, Mikhail, K. Lehmann, and M. Wittke. 2018. “Robo Advisors: Quantitative Methods inside the Robots.” Journal of Asset Management 19 (6): 363–70. https://doi.org/10.1057/s41260-018-0092-9.
Belanche, Daniel, L. Casaló, M. Flavián, and S. Correia Loureiro. 2023. “Benefit versus Risk: A Behavioral Model for Using Robo-Advisors.” Service Industries Journal February: 1–28. https://doi.org/10.1080/02642069.2023.2176485.
Better Finance. 2021, January 25. “Robo-Advice 5.0: Can Consumers Trust Robots?” Better Finance. https://betterfinance.eu/.
Bianchi, Milo, and M. Briere. 2021. “Robo-Advising: Less AI and More XAI?” Available at SSRN 3825110.
BlackRock. 2016. “Digital Investment Advice: Robo-Advisors Coming of Age.” BlackRock. www.professionalplanner.com.au/wp-content/uploads/2016/09/viewpoint-digital-investment-advice-robo-sep-2016_Aust.pdf.
Boston Consulting Group. 2023, May 15. “Global Asset Management Industry Must Transform to Thrive amidst Changing Macroeconomics.” BCG Global. www.bcg.com/press/15may2023-global-asset-management-transform-to-thrive.
Brenner, Lukas, and T. Meyll. 2020. “Robo-Advisors: A Substitute for Human Financial Advice?” Journal of Behavioral and Experimental Finance 25 (2020): 100275.
Brown, Mike. 2017, September 19. “Millennials: Robo-Advisors or Financial Advisors?” LendEDU (blog). https://lendedu.com/blog/robo-advisors-vs-financial-advisors/.
Campbell, Donald Thomas, and J. Stanley. 1963. Experimental and Quasi-Experimental Designs for Research.
CFI. 2023. “Robo-Advisors.” Corporate Finance Institute. https://corporatefinanceinstitute.com/resources/wealth-management/robo-advisors/.
Chen, Mark A, Q. Wu, and B. Yang. 2019. “How Valuable Is Fintech Innovation?” Review of Financial Studies 32 (5): 2062–2106. https://doi.org/10.1093/rfs/hhy130.
Cheng, Xusen, F. Guo, J. Chen, K. Li, Y. Zhang, and P. Gao. 2019. “Exploring the Trust Influencing Mechanism of Robo-Advisor Service: A Mixed Method Approach.” Sustainability (2071-1050) 11 (18): 4917. https://doi.org/10.3390/su11184917.
Creswell, John W., and C. Poth. 2018. Qualitative Inquiry & Research Design: Choosing among Five Approaches. 4th ed. Thousand Oaks, California: SAGE.
D’Acunto, Francesco, and A. Rossi. 2022, October 5. “Robo-Advice: An Effective Tool to Reduce Inequalities?” Brookings. www.brookings.edu/articles/robo-advice-an-effective-tool-to-reduce-inequalities/.
de Jesus, Ayn. 2021, July 2. “Robo-Advisors and Artificial Intelligence—Comparing 5 Current Apps.” Emerj Artificial Intelligence Research. https://emerj.com/ai-application-comparisons/robo-advisors-artificial-intelligence-comparing-5-current-apps/.
Deloitte. 2016. “Robo-Advisory in Wealth Management.” Deloitte Consulting. www2.deloitte.com/content/dam/Deloitte/de/Documents/financial-services/Robo_No_2.pdf.
D’Hondt, Catherine, R. De Winne, E. Ghysels, and S. Raymond. 2020. “Artificial Intelligence Alter Egos: Who Might Benefit from Robo-Investing?” Journal of Empirical Finance 59 (December): 278–99. https://doi.org/10.1016/j.jempfin.2020.10.002.
Dietvorst, Berkeley J., J. Simmons, and C. Massey. 2015. “Algorithm Aversion: People Erroneously Avoid Algorithms after Seeing Them Err.” Journal of Experimental Psychology: General 144 (1): 114–26. https://doi.org/10.1037/xge0000033.
Doherty, Dana, and K. Curran. 2019. “Chatbots for Online Banking Services.” Web Intelligence (2405-6456) 17 (4): 327–42. https://doi.org/10.3233/WEB-190422.
Fisch, J. E., M. Laboure, and I. Turner. 2019. “The Emergence of the Robo-Advisor.” In The Disruptive Impact of Fintech on Retirement Systems: 13–37. Pension Research Council Series. Oxford: Oxford University Press.
Foerster, Stephen, J. Linnainmaa, B. Melzer, and A. Previtero. 2017. “Retail Financial Advice: Does One Size Fit All?” Journal of Finance 72 (4): 1441–82. https://doi.org/10.1111/jofi.12514.
Friedberg, Barbara, and B. Curry. 2022, July 8. “Top-10 Robo-Advisors by Assets (AUM) – Forbes Advisor.” Forbes Advisor. www.forbes.com/advisor/investing/top-robo-advisors-by-aum/.
Grable, John, and R. Lytton. 1999. “Financial Risk Tolerance Revisited: The Development of a Risk Assessment Instrument.” Financial Services Review 8 (3): 163. https://doi.org/10.1016/S1057-0810(99)00041-4.
Guo, Fei, X. Cheng, and Y. Zhang. 2019. “A Conceptual Model of Trust Influencing Factors in Robo-Advisor Products: A Qualitative Study.” WHICEB 2019 Proceedings June. https://aisel.aisnet.org/whiceb2019/48.
High Level Expert Group on AI. 2019. “Ethics Guidelines for Trustworthy AI—Shaping Europe’s Digital Future.” European Commission Report. Brussels. https://digital-strategy.ec.europa.eu/en/library/ethics-guidelines-trustworthy-ai.
Hildebrand, Christian, and A. Bergner. 2021. “Conversational Robo Advisors as Surrogates of Trust: Onboarding Experience, Firm Perception, and Consumer Financial Decision Making.” Journal of the Academy of Marketing Science 49 (4): 659–76. https://doi.org/10.1007/s11747-020-00753-z.
HSBC. 2019. “Trust in Technology.” UK: HSBC. www.hsbc.com/-/files/hsbc/media/media-release/2017/170609-updated-trust-in-technology-final-report.pdf.
Huang, Zhuo. 2022. “Robo-Advisors and the Digitization of Wealth Management in China.” In The Digital Financial Revolution in China. Washington, D.C.: The Brookings Institution.
Investment Company Institute. 2022. “Investment Company Fact Book.” Trends and Activities. Washington, D.C.: Investment Company Institute. www.ici.org/fact-book.
Investment Company Institute. 2023. “ICI Research Perspective: Trends in the Expenses and Fees of Funds, 2022.” Research. Washington, D.C.: Investment Company Institute. www.ici.org/system/files/2023-03/per29-03.pdf.
Isaia, Eleonora, and N. Oggero. 2022. “The Potential Use of Robo-Advisors among the Young Generation: Evidence from Italy.” Finance Research Letters 48 (1).
Jacovi, Alon, A. Marasović, T. Miller, and Y. Goldberg. 2021. “Formalizing Trust in Artificial Intelligence: Prerequisites, Causes and Goals of Human Trust in Ai.” arXiv. https://doi.org/10.48550/arXiv.2010.07487.
Jarek, Krystyna, and G. Mazurek. 2019. “Marketing and Artificial Intelligence.” Central European Business Review 8 (2): 46–55. https://doi.org/10.18267/j.cebr.213.
Jones, Gareth R., and J. George. 1998. “The Experience and Evolution of Trust: Implications for Cooperation and Teamwork.” Academy of Management Review 23 (3): 531–46. https://doi.org/10.5465/AMR.1998.926625.
Kaplan, Alexandra D., T. Kessler, J. Christopher Brill, and P. A. Hancock. 2023. “Trust in Artificial Intelligence: Meta-Analytic Findings.” Human Factors 65 (2): 337–59. https://doi.org/10.1177/00187208211013988.
Kim, Hee-Woong, Y. Xu, and J. Koh. 2004. “A Comparison of Online Trust Building Factors between Potential Customers and Repeat Customers.” Journal of the Association for Information Systems 5 (10): 392–420.
Kitces, Michael. 2017, December 19. “What Robo-Advisors Can Teach Human Advisors.” Nerd’s Eye View [blog]. www.kitces.com/blog/dan-egan-betterment-podcast-robo-advisor-evidence-based-behavioral-finance-research/.
Linnainmaa, Juhani T., B.T. Melzer, and A. Previtero. 2020. “The Misguided Beliefs of Financial Advisors.” The Journal of Finance 76 (2): 587–621.
Lui, Alison, and G. Lamb. 2018. “Artificial Intelligence and Augmented Intelligence Collaboration: Regaining Trust and Confidence in the Financial Sector.” Information & Communications Technology Law 27 (3): 267–83. https://doi.org/10.1080/13600834.2018.1488659.
Maume, Philipp. 2021. Robo-Advisors How Do They Fit in the Existing EU Regulatory Framework, in Particular with Regard to Investor Protection? Luxembourg: European Parliament. https://doi.org/10.2861/88874.
McKnight, D. Harrison, L. Cummings, and N. Chervany. 1998. “Initial Trust Formation in New Organizational Relationships.” Academy of Management Review 23 (3): 473–90. https://doi.org/10.5465/AMR.1998.926622.
Melián-González, Santiago, D. Gutiérrez-Taño, and J. Bulchand-Gidumal. 2021. “Predicting the Intentions to Use Chatbots for Travel and Tourism.” Current Issues in Tourism 24 (2): 192–210. https://doi.org/10.1080/13683500.2019.1706457.
Nashold, D. Blaine. 2020. “Trust in Consumer Adoption of Artificial Intelligence-Driven Virtual Finance Assistants: A Technology Acceptance Model Perspective.” Doctoral dissertation. Charlotte, North Carolina: The University of North Carolina at Charlotte. http://search.proquest.com/docview/2385705772/abstract/56B751DCB9834E1CPQ/3.
Niszczota, Paweł, and D. Kaszás. 2020. “Robo-Investment Aversion.” PLoS ONE 15 (9): 1–19. https://doi.org/10.1371/journal.pone.0239277.
Nunnally, Jum C. 1978. Psychometric Theory. 2nd ed. McGraw-Hill series in psychology. New York: McGraw-Hill.
Oshima, T.Chris, and T. Dell-Ross. 2016. All Possible Regressions Using IBM SPSS: A Practitioner’s Guide to Automatic Linear Modeling. Digital Commons: Georgia Southern University.
Peterson, Robert A. 1994. “A Meta-Analysis of Cronbach’s Coefficient Alpha.” Journal of Consumer Research 21 (2): 381–91. https://doi.org/10.1086/209405.
Prahl, Andrew, and L. Van Swol. 2017. “Understanding Algorithm Aversion: When Is Advice from Automation Discounted?” Journal of Forecasting 36 (6): 691–702. https://doi.org/10.1002/for.2464.
Securities and Exchange Commission. 2017. “Investor Bulletin: Robo-Advisers.” www.sec.gov/oiea/investor-alerts-bulletins/ib_robo-advisers.
Sheridan, Thomas B. 2019. “Individual Differences in Attributes of Trust in Automation: Measurement and Application to System Design.” Frontiers in Psychology 10 (May). https://doi.org/10.3389/fpsyg.2019.01117.
Sironi, Paolo. 2016. FinTech Innovation: From Robo-Advisors to Goal Based Investing and Gamification. Brisbane, United Kingdom: John Wiley & Sons, Incorporated. http://ebookcentral.proquest.com/lib/jwu/detail.action?docID=4603179.
Statista. 2023. “U.S.: Robo-Advisors AUM 2017-2027.” www.statista.com/forecasts/1259591/robo-advisors-managing-assets-united-states.
Thier, Christian, and D. dos Santos Monteiro. 2022. “How Much Artificial Intelligence Do Robo-Advisors Really Use?” SSRN Scholarly Paper. Rochester, NY. https://doi.org/10.2139/ssrn.4218181.
Trecet, Jose. 2019, April 1. “Un Robot a Cargo de Tus Inversiones: Así Funcionan los Robo Advisors.” Business Insider España. www.businessinsider.es/como-funciona-robo-advisor-como-saber-ti-388261.
Wells Fargo. 2016. “Wells Fargo/Gallup Survey: Investors Curious about Digital Investing, More Optimistic about Economy Prior to Brexit.” Wells Fargo Newsroom. https://newsroom.wf.com/English/news-releases/news-release-details/2016/Wells-FargoGallup-Survey-Investors-Curious-About-Digital-Investing-More-Optimistic-About-Economy-Prior-to-Brexit/default.aspx.
Yang, Xue. 2019. “How Perceived Social Distance and Trust Influence Reciprocity Expectations and eWOM Sharing Intention in Social Commerce.” Industrial Management & Data Systems 119 (4): 867–880.